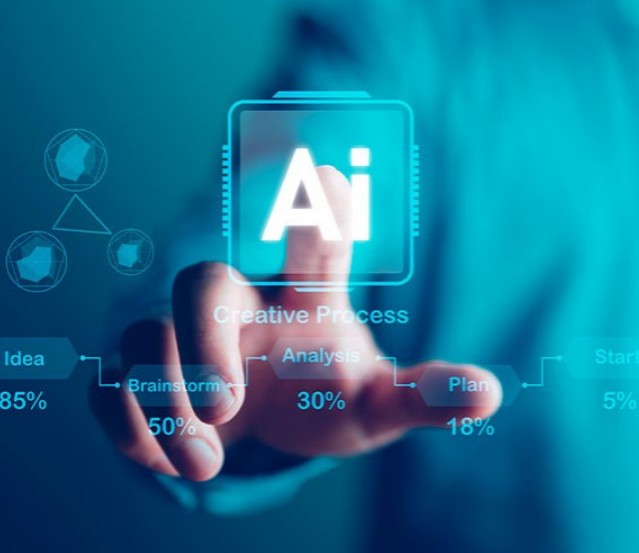
In just a few years, generative AI has gone from futuristic novelty to daily research companion. It transcribes interviews, summaries readings, codes data — sometimes before you’ve finished your coffee.
But as qualitative researchers, we need to ask: is AI deepening our insights or flattening them? Can it enhance our practice without compromising what makes qualitative research meaningful?
I’ve tested over a number of tools across real-world projects. Some have been game-changing, others frustrating or even misleading. This post breaks down what AI can do, what it can’t, and why human judgement remains irreplaceable.
What AI Can Do: Speed, Structure, Support
Used thoughtfully, AI offers real benefits across a number of research areas:
Transcription and Summarisation
AI-powered tools like Whisper, Otter.ai, or Gemini can transcribe audio and summarise key themes in minutes. This alone saves hours of manual labour.
Literature Reviews
AI can synthesise findings from multiple papers. Acheampong and Nyaaba (2024) show that AI supports topic mapping, summary writing, and even detecting research gaps — if prompts are well designed.
Theme Clustering and Coding Support
Studies like Carvalho et al. (2024) and Zhang et al. (2023) find that AI can reliably identify dominant themes in open-ended data — especially with strong prompting. Tools like QualiGPT or ChatGPT-4 can surface initial codes, suggest categories, and generate summaries. It’s like having a fast assistant for early-stage analysis.
What AI Can’t Replace: Interpretation, Reflexivity, Ethics
Despite the hype, AI has serious limitations. It doesn’t interpret meaning. It doesn’t understand your participants. And it certainly doesn’t do reflexivity.
Interpretation Is Human Work
AI detects patterns. It doesn’t understand contradiction, metaphor, or lived experience. As we know, and also highlighted in recent studies on the role of AI in qualitative research (e.g., Christou, 2023 & Hitch, 2024), qualitative research is about depth, context, and meaning, not frequency.
Reflexivity Is Not Programmable
AI cannot reflect on power, identity, or its own assumptions; it has no positionality, no standpoint. As qualitative researchers, this kind of critical reflexivity is our responsibility, not something that can be outsourced to an algorithm.
Ethical Awareness Requires Oversight
AI can hallucinate citations, reproduce bias, and generate content that sounds right but is wrong. Roberts et al. (2024) caution that LLMs imitate language patterns, not truth, with AI’s ‘human like’ qualities resulting from statistical predictions and not comprehension. Without human review, misleading or unethical outputs can slip through.
The Ethical Dilemma: Speed Versus Depth
So, what’s the real danger?
It’s not that AI is ‘bad.’ It’s that it’s convincing. A fluent, confident-sounding AI summary can lull researchers into skipping the hard work — interpretation, contextualisation, accountability. And without transparency, readers may never know that parts of the analysis were machine-generated.
Key risks include:
- Bias replication from skewed training data (Acheampong and Nyaaba, 2024)
- Fabricated citations in AI-generated literature reviews (Christou, 2023a)
- Over simplified coding that misses emotional or marginal perspectives (Carvalho et al., 2024)
When to Use AI — And Where Human Insight Remains Essential
Task | AI Can Help With | Human Role |
Transcription | Speed, efficiency, capturing most words accurately | Review for nuance, emotion, accents, errors and ethical compliance |
Literature Summarisation | Drafting summaries, clustering themes | Verify sources, assess argument quality, ensure citation accuracy |
Theme Identification | First-pass clustering, detecting common patterns | Refine codes, interpret meaning, surface less obvious insights |
In-depth Analysis | Highlighting patterns, assisting with comparisons | Theoretical framing, contextualisation, reflexive interpretation |
Theory Development | Suggesting connections or clusters of ideas | Abstraction, synthesis, grounding in field knowledge |
Ethics & Reflexivity | Raising initial flags (e.g., sensitive language detection) | Ensure transparency, positionality, and reflexivity |
Final Thoughts: Use AI as a Mirror, not a Crutch
AI can speed things up. It can help you notice patterns. But it can’t replace the human work of interpretation, theory-building, and ethical decision-making.
As researchers, we’re not here to outsource thinking, we’re here to do it better. AI can absolutely help, but we need to stay in the driver’s seat.
Explore further in this upcoming free webinar!
Curious but cautious about using AI in your research?
Join my upcoming free 1-hour webinar, where we’ll explore in more detail
- What AI actually does in qualitative research
- Where it goes wrong — and why that matters
- How to think ethically, not just efficiently
Webinar: AI in Qualitative Research: Thoughtful, Ethical, and Effective Use
A practical session for researchers exploring or applying AI in qualitative projects
Date: April 17, 2025 | 11am AEST
Register here
References
Acheampong, K. O., & Nyaaba, M. (2024). Review of qualitative research in the era of generative artificial intelligence. Journal of Empirical Research on Human Research Ethics, 19(3), 92-102.
Carvalho, T., Negm, H., & El-Geneidy, A. (2024). A Comparison of the Results from Artificial Intelligence-based and Human-based Transport-related Thematic Analysis. Findings.
Christou, P. A. (2023). How to use artificial intelligence (AI) as a resource, methodological and analysis tool in qualitative research? The Qualitative Report, 28(7), 1968-1980.
Hitch, D. (2024). Artificial intelligence augmented qualitative analysis: The way of the future? Qualitative Health Research, 34(7), 595-606.
Roberts, J., Baker, M., & Andrew, J. (2024). Artificial intelligence and qualitative research: The promise and perils of large language model (LLM) ‘assistance’. Critical Perspectives on Accounting, 99, 102722.
0 Comments